π¬ Lab 1: Least Squares#
Before starting this lab, please review the following tutorials: Python Basic, Python Intermediate, and Numpy.
Python Basic: While you may already be familiar with much of this material, itβs recommended to review it as a refresher.
Python Intermediate: Go through this tutorial carefully. Many past ECE487 cadets lacked familiarity with the topics covered here.
NumPy: We will be using NumPy extensively in this class. A solid understanding of this tutorial is highly recommended.
Least Sqaures Estimation#
In this lab, we will solve a least squares regression problem to find the best-fit linear equation for a set of measured data. Let the measured data be represented by \(\mathbf{t} = (t_1, t_2, \cdots, t_n)\), with corresponding input values \(\mathbf{x} = (x_1, x_2, \cdots, x_n)\).
Our goal is to find \(w_1\) and \(w_0\) such that the equation \(t = w_1x + w_0\) best approximates the linear relationship between \(x\) and \(t\).
Plotting the Data#
First, letβs visualize the data:
import numpy as np
import matplotlib.pyplot as plt
x = np.array([0, 1, 2, 3])
t = np.array([-1, 0.4, 0.7, 2.1])
fig = plt.figure(figsize=(4, 3))
ax = fig.add_subplot()
plt.plot(x, t, "o", markersize=5)
plt.xlabel("x")
plt.ylabel("t")
plt.grid(True)
plt.show()
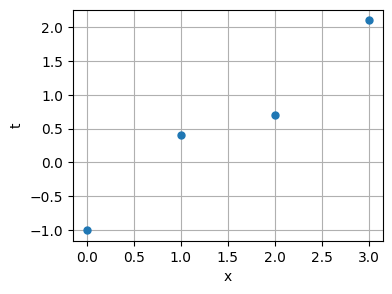
Linear Equation in Matrix Form#
We can express the linear equation in matrix form as \(t = \mathbf{c} \cdot \mathbf{w}\), where:
\(\mathbf{c} = \begin{bmatrix} x & 1 \end{bmatrix}\) and \(\mathbf{w} = \begin{bmatrix} w_1 \ w_0 \end{bmatrix}\). This gives us:
\(t = w_1x + w_0 = \begin{bmatrix} x & 1 \end{bmatrix} \begin{bmatrix} w_1 \ w_0 \end{bmatrix} = \mathbf{c} \cdot \mathbf{w}\).
Given \(\mathbf{x} = (x_1, x_2, \cdots, x_n)\) and \(\mathbf{t} = (t_1, t_2, \cdots, t_n)\), we can set up the following system of equations:
\(\begin{bmatrix} -1 \ 0.4 \ 0.7 \ 2.1 \end{bmatrix} = \begin{bmatrix} 0 & 1 \ 1 & 1 \ 2 & 1 \ 3 & 1 \end{bmatrix} \begin{bmatrix} w_1 \ w_0 \end{bmatrix}\),
or, in matrix notation, \(\mathbf{t} = \mathbf{C}\mathbf{w}\).
Here:
\(\mathbf{C} = \begin{bmatrix} x_1 & 1 \ x_2 & 1 \ x_3 & 1 \ x_4 & 1 \end{bmatrix} = \begin{bmatrix} 0 & 1 \ 1 & 1 \ 2 & 1 \ 3 & 1 \end{bmatrix}\).
Creating the Matrix \(\mathbf{C}\)#
We can create the \(C\) matrix using np.column_stack
ones = np.ones_like(x) # Create an array of ones with the same shape as x
C = np.column_stack((x, ones)) # Combine x and ones as columns
print(C)
[[0 1]
[1 1]
[2 1]
[3 1]]
Solving for \(\mathbf{w}\) Using np.linalg.lstsq#
Next, we can use the np.linalg.lstsq function to solve for \(\mathbf{w}\):
w = np.linalg.lstsq(C, t, rcond=None)[0]
print(w)
# Or you can extract the individual coefficients
w1, w0 = np.linalg.lstsq(C, t, rcond=None)[0]
print(w1, w0)
[ 0.96 -0.89]
0.9599999999999999 -0.8899999999999998
With \(w_1 = 0.96\) and \(w_0 = -0.89\), the best-fitting line is:
\(t = 0.96x - 0.89\)
Plotting the Fitted Line#
Now, letβs plot the fitted line alongside the data points:
fig = plt.figure(figsize=(4, 3))
ax = fig.add_subplot()
plt.plot(x, t, "o", label="Measurements", markersize=5)
plt.plot(x, w1 * x + w0, "r", label="Fitted Line")
plt.xlabel("x")
plt.ylabel("t")
plt.legend()
plt.grid(True)
plt.show()
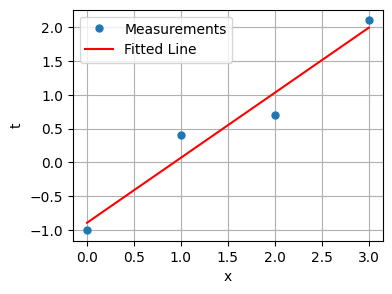
π» Procedure#
Update Your Code#
Navigate to the ECE487 workspace,
ece487_wksp
.Right-click on the
ece487_wksp
folder and chooseGit Bash Here
from the menu.To pull the latest updates, run the following command:
git pull upstream main
Galileiβs Falling Body Experiment#
Open the
falling_body.ipynb
notebook in VS Code.Carefully review the contents of this notebook to understand the three different techniques used for curve fitting.
Deliverables#
Open the
Lab1_LSE.ipynb
notebook using VS Code.Follow the instructions within the notebook to complete your code.
Push your code to your GitHub repository and submit the output plot in Gradescope.